Table of Contents
1. Introduction
Customer Data Platforms (CDPs) are a technology that has become popular in recent years primarily due to the need to leverage both marketing and business data. We can summarize these needs in the following points:
- Activate First Party Data: Companies collect a lot of information from their customers, but lack the necessary tools to make use of this data. Activating the information is an effective way to get greater results, especially in market categories where data intelligence is highly profitable.
- Adapting to the cookieless future: With the growing impact of new privacy measures on search engines and devices and the upcoming end of third party cookie support in Chrome leads companies to seek new technologies to optimize their marketing efforts through personalization.
- Breaking information silos: Large companies have been collecting data for years, but all this information is distributed in silos, making it impossible to analyze at scale, heuristically and with greater impact on the organization’s decisions.
- Complying with regulatory frameworks: Governments around the world are increasingly forcing all types of companies to comply with ever stricter legal frameworks around consumers’ personal data. Processing personal data is already a process that requires strict layers of data governance and compliance with user consent.
- Improving the customer experience: Customers are increasingly looking for two fundamental aspects, that their privacy is respected and at the same time that they are granted an appropriate level of personalization. Both requests, although contradictory in themselves, represent a strategic and technological challenge.
These 5 needs can be met by a CDP, which provides the tools and capabilities needed to manage, analyze and action customer data in a way that not only meets regulatory requirements, but also enables greater personalization and improves the customer experience. With a unified view of customer data, organizations can break down information silos, adapt to a cookie-less future and activate their First Party Data to gain actionable insights and create more effective marketing and business strategies.
This article exists for the purpose of providing all the information necessary to understand the relevance, functionality and advantages that Customer Data Platforms (CDPs) can provide in today’s digital environment. It will address how CDPs enable organizations to effectively activate and leverage their First Party Data, adapt to the changing data privacy landscape, and break down information silos that can hinder a 360 view of the customer. It will also explore how CDPs can help companies stay compliant with increasingly stringent regulatory frameworks while simultaneously improving the customer experience through well-rounded personalization. Valuable and practical insights will also be provided on how to select, implement and optimize a CDP to maximize ROI and align marketing and business efforts with customer expectations and needs in the digital age.
1.1 Definition of CDP
The CDP Institute is one of the most prominent sources of information, they propose a definition of CDP quite simple: “CDP is packaged software that creates a persistent, unified customer database that is accessible by other systems”. In addition to that they mark 4 different types of CDP: Data, Analytics, Campaigns and Distribution CDPs.
Many other sources such as Oracle and Martech.org share in one way or another this same definition. However, these definitions do not include other fundamental aspects of CDPs, such as governance, the possibility of having composable CDPs or even the possible roles that this technology may have in a modern data technology stack. In our opinion, the definition of CDP should be somewhat more complete:
A CDP (Customer Data Platform) is a technology platform, either packaged or composable, whose role is to break down information silos (from different sources) associated with customer information through customer identity resolution. The functional objective of a CDP is to integrate, activate, transform and facilitate all customer data through a robust governance and privacy layer.
It should be clarified that a CDP, depending on its composition and location in a technology stack, may represent a new data silo in itself (a criticism that other industry experts have also highlighted). This occurs because within any large organization there are already other resources that fulfill part of the functions that a CDP fulfills. This overlapping of functions easily leads to confusion in which one questions how a CDP should function, or indeed questions whether it is a truly necessary technology.
Despite these criticisms, the reality is that CDPs offer a more focused and specialized customer data management solution with a strong self-service layer usable by non-technical profiles within an organization, facilitating a unified and accessible view of data that can be instrumental in improving marketing strategies and personalizing the customer experience. In addition, CDPs excel in their ability to resolve customer identities across different touch points and channels, providing a unified source of truth that is essential for an accurate and complete understanding of the customer.
The modular and flexible nature of CDPs also allows for easier integration with other systems and technologies in an organization’s technology stack, thus providing a more cohesive and efficient data architecture. Data governance and privacy are other critical aspects that CDPs are designed to handle efficiently, helping organizations comply with evolving data privacy regulations and ensure the protection of customer data. These functions although perfectly mimicable by other technologies would require a great deal of development and engineering effort to achieve, whereas with a CDP such functions are simply developed and working.
Considering the above, there are two main groups of CDPs, packaged and composable.
1.1.1 Packaged CDP
A packaged CDP is a customer data management solution that is offered as a complete, out-of-the-box product. This type of CDP comes with a predefined set of features and functionality that are designed to help organizations capture, manage, analyze and activate customer data. Consequently the amount of IT and engineering resources required are considerably less compared to a composable CDP. The key features of a packaged CDP are:
- Predefined Structure: They have a predefined data structure and architecture designed to satisfy common customer data management needs. This does not imply that they lack the flexibility to adapt to different information structures.
- Ready-to-use functionalities: They offer a set of advanced functionalities that are already ready to operate such as data integration, customer identity resolution, customer segmentation, advanced analytics, audience orchestration, governance, consent and privacy management, etc.
- Friendly User Interface: They provide intuitive user interfaces and preconfigured dashboards that facilitate data visualization and management and allow teams with little technical experience to extract maximum value from customer data.
- Quick Implementation: They are designed for quick and easy implementation, allowing the platform to be up and running in a reduced time. This also reduces the need to hire specialized technical personnel.
- Pre-built integrations: Include pre-built integrations with other common systems and platforms, facilitating the connection with other parts of the technological stack of an organization, saving maintenance costs.
- Basic Regulatory Compliance: They provide basic features to help organizations comply with data privacy regulations and other legal requirements.
- Maintenance and Support: Bundled CDP vendors typically offer support and maintenance as part of the package, ensuring access to technical help when needed.
- Cost-Effectiveness: By having a predefined structure and functionalities, they can be more cost-effective compared to customized or composable solutions.
- Training and Documentation: They usually come with full documentation and training options to help users understand and use the platform effectively.
- Time Optimization: By reducing the need for extensive development and configuration, they allow organizations to optimize their time and resources.
- Scalability: Depending on the vendor they may have limited scalability compared to composable CDPs, however it is rare that a packaged CDP cannot handle thousands or even millions of records with low latency time.
The main advantage of a packaged CDP is that it is easier and faster to implement, as it comes with a default structure and configuration that has been designed to address common customer data management needs. This can include functionality such as data integration, identity resolution, customer segmentation, analytics and data activation for marketing campaigns.
This also includes features that are normally omitted, such as a friendly and easy-to-use user interface with the objective of enabling different members of the organization to
Organizations that opt for a packaged CDP typically do so because they are looking for a standard solution that can address their customer data management needs effectively, without the need for extensive configuration or development. This can be especially beneficial for organizations with limited resources or technical expertise, or those looking for a quick solution to address their customer data challenges.
A packaged CDP provides a plug-and-play solution for customer data management, with a predefined architecture and set of functionalities that are ready to use with little or no customization required.
Among the best-known solutions on the market are:
Name | Country | Revenue | Employees | Foundation |
---|---|---|---|---|
Treasure Data | United States | 100.5M (est) | 600+ | 2011 |
Salesforce | United States | 33.07B (est) | 10.000+ | 1999 |
Adobe | United States | 15.8B (est) | 29.239 | 1982 |
Segment | United States | 108.3M (est) | 584 | 2011 |
Zeotap | Germany | 32.5M (est) | 216 | 2014 |
Tealium | United States | 116.5M (est) | 655 | 2008 |
Snowcat Cloud | United States | N/A | 1 | 2020 |
In other words, a packaged CDP is a ready-to-run solution where the only effort required is its integration with the current technology stack and the onboarding of the work team (knowledge transfer, change management and process optimization). This naturally has implications that we will see later on.
1.1.2 Composable CDP
A composable CDP, as opposed to a packaged CDP, is a more flexible and customizable solution for customer data management. This variant of the platform allows for deeper configuration and adaptation to an organization’s specific needs, offering a modular structure that can be designed to address challenges that are specialized and for which no packaged CDP can easily address.
Key features of a composable CDP include:
- Custom Configuration: Composable CDPs allow for custom configuration according to the organization’s needs, providing the flexibility to adapt to specific data management requirements, this is especially useful in highly complex data structures or in an advanced state of maturity and quality.
- Custom Development: They offer the possibility of having a customized development, which allows organizations to build and add functionalities according to their more specialized requirements. This is obviously an advantage in those organizations with a high availability of engineering resources and technical knowledge.
- Deep Integration: They allow a deep integration with the company’s technological infrastructure, which has a profound impact on the cost of servers and data transfer, in addition to having the opportunity to substantially reduce data latency.
- Advanced Scalability: Composable CDPs provide advanced and fully customized scalability, allowing organizations to grow and expand their data management capabilities as their needs evolve, or even reduce the amount of resources in those operations that do not require excessively high scalability.
- Advanced Data Governance: They include advanced capabilities for data governance, allowing a more rigorous management of regulatory compliance and data privacy. In addition, by having all customer data in its own infrastructure without relying on a third party (as would be the case in a packaged CDP), different security risks are mitigated.
- Advanced Data Analytics and Activation: They offer advanced capabilities for data analysis and activation, providing powerful tools to extract valuable insights and drive data-driven marketing and business strategies. In addition, as a composable solution, it allows teams to use business intelligence tools that are already available in the organization.
- Reduced overlap: As a fully customizable solution, it is possible for organizations to determine what is necessary, and what is not. This results in less overlap between tools and at the same time reduces the budget waste inherent in these cases.
Organizations that opt for a composable CDP generally have more complex data management, activation and governance needs, and value the ability to customize and tailor the platform to their specific processes and challenges. This may require a larger upfront investment, both in time and resources, for platform configuration, development, integration and maintenance. However, the result is a robust and tailored solution that can provide significant value over time.
This also brings an implicit risk of composable solutions: being a maintenance dependent on the company and its employees, it runs a greater risk of becoming obsolete in the face of the accelerated change we are experiencing in areas such as data activation in marketing. Unlike a packaged CDP, where there are hundreds of people working to keep a technological solution current and competitive.
It is crucial to understand that a composable CDP does not necessarily require all of its functions to be fully custom-developed. Commonly, in developing a composable CDP, organizations choose to contract various technology tools, integrating them into their existing technology infrastructure, in order to gain flexibility and minimize development investment. For example, an organization might opt for a composable CDP that incorporates a “Reverse ETL” tool for data activation and a “Business Intelligence” tool for customer data analysis, while choosing to custom-develop the customer identification resolution service. That said, there are vendors geared to offer CDP solutions specialized in composable CDP:
Name | Country | Revenue | Employees | Foundation |
---|---|---|---|---|
Hightouch | United States | 29.1M (est) | 187 | 2018 |
Census | United States | 31.6M (est) | 210 | 2018 |
Snowplow | England | N/A | 156 | 2012 |
Rudderstack | United States | 30.9M (est) | 198 | 2019 |
A composable CDP requires considerable effort in integration with the existing technology stack, as well as team onboarding (knowledge transfer, change management and process optimization). However, this initial effort can result in a customer data management solution that is highly effective and aligned with the organization’s strategic objectives.
In the following section, we will explore the implications and considerations to take into account when opting for a composable CDP, as well as strategies to maximize the value and impact of this advanced technology in the organization’s data strategy.
1.1.3 Comparison: composable vs. packaged
In the realm of customer data management, Customer Data Platforms (CDPs) emerge as crucial tools that enable organizations to consolidate and activate data to improve decision making and optimize marketing strategies. However, not all CDPs are created equal, and it is essential to understand the fundamental differences between packaged and composable variants to choose the one that best fits an organization’s needs and capabilities.
A packaged CDP is characterized by offering an out-of-the-box solution with predefined functionalities that facilitate customer data management and analysis. On the other hand, a composable CDP provides a more flexible and customizable approach, allowing for tailored configuration to address specific data challenges.
In the following table, we present a detailed comparison between these two types of CDP, covering various aspects such as configuration, development, flexibility, implementation, cost, data governance, and other crucial factors that may influence the choice between one or the other CDP model. This comparison aims to provide a clear view of the advantages and disadvantages of each type, thus facilitating informed decision-making in the adoption of a platform that will enhance an organization’s data strategy.
Feature | Packaged CDP | Composable CDP |
---|---|---|
Configuration | Predefined by the vendor, flexible. | Fully customizable. |
Development | Fully outsourced to software vendor. Requires investment in implementation. | Tailor-made, it requires a previous technical design phase. |
Identity resolution | They have pre-built tools specialized in recognizing a single user through multiple identifiers. | They usually rely on existing data joins in the data warehouse, or additional data structures need to be developed to accomplish this task. Usually a mature company already has this challenge solved and the composable CDP can use these existing solutions. |
Flexibility | Limited. | 100% flexible. |
Implementation | Quick and relatively straightforward. 6 months of project is a reasonable benchmark. | More Complex and time and development intensive.12 months of project is a reasonable benchmark. |
Integrations | Pre-built and maintained by the vendor. | Customized or pre-built, depending on development decisions. |
Cost | Generally lower | Generally higher |
Maintenance and Support | Provided by the seller. | Requires a specialized in-house team or a technology partner. |
Scalability | Predefined by the characteristics of the contracted service | Completely flexible and dependent on the existing technology stack |
Data Governance | Advanced and ready to use. They usually rely on a complete copy of the required data in the CDP and therefore have a specialized control layer already developed. | Depends on the design and infrastructure in place. They usually have the data warehouse at the center of the infrastructure, which allows for greater flexibility and granular control. |
Data Analysis | Advanced functionalities pre-established by the vendor. | They usually have to be outsourced to specialized software. |
Data Activation | It has all the most common necessary functions. | Customizable according to business needs. |
Training and Documentation | Provided by the seller. | It falls on the development team. |
Time Optimization | High, as it is faster to implement. | Low, as it requires a lot of custom work. |
Latency | Being an external infrastructure, it has a higher risk of presenting data latency. On the other hand, they already have infrastructure ready to perform certain tasks in “real-time”, an aspect that is not usual to find in a composable CDP. | In a well-designed model, latency is minimized by relying on the same infrastructure of the organization. However, the technological cost of achieving real-time integrations can be a challenge. |
Technological overlap | Greater, by having functions and tools that supplement other technologies already contracted. | Lower, as it is possible to reuse technologies already contracted. |
Risks | Vendor dependency. Vendor lock-in. Arbitrary changes in license cost. | High maintenance costs. Fast obsolescence without the necessary maintenance investment. A higher time-to-value. |
Benefits | Easy implementation and use. Much lower time-to-value. Native integration in some ecosystems such as Adobe. | Customization and flexibility. Less duplication of data. Less risk of vendor lock-in. |
Certainly there are open questions and detailed analyses that allow to better analyze the difference between composable and packaged. In any case, the most important thing is to understand the technological and business needs: What are the use cases to be solved, what data is currently available, what is the current technological maturity and how much talent does the organization have.
Of course, deciding between a composable or packaged CDP is not a simple decision, but ultimately it is a completely objective decision. To this end, it is important to consider the various factors that may lead an organization to opt for one of the two. To facilitate this process we have prepared this simple questionnaire that can help to resolve this question at a high level.
1.2 Importance of CDPs in the Business Strategy
The relevance of Customer Data Platforms (CDP) in business strategy is indisputable in the current era, where data activation through audiences, personalization of buying journeys or even a better understanding of the business is key. The ability to activate data has become a key competitive differentiator. Inactive data equals lost opportunity, and this is where CDPs play a critical role, transforming raw data into actionable insights. Here are the main areas where a CDP has an impact at the business level:
Customer Data Unification: CDPs remove the barriers between the different data sources present in an organization, unifying customer information into a single view regardless of whether the customer has different identifiers across different sources. This holistic view is vital to fully understand the customer journey and to deliver personalized, consistent, high-quality experiences.
Orchestration of Data Flows: Efficient orchestration of data flows across various platforms and channels is essential so that the unification of customer data can be exploited across different modeled data. CDPs enable this orchestration, resulting in smarter and more timely interactions with customers.
Personalization at Scale: In a market where personalization is not only desired but expected by consumers, CDPs enable companies to deliver highly personalized offers and communications at scale with low levels of latency (which is a considerable technical challenge). This capability improves customer retention and increases conversion rates.
Data Privacy Compliance: With increasing data privacy regulations, CDPs offer a solution by providing a framework that respects user consent and ensures compliance with data protection laws such as GDPR and CCPA.
Data-Driven Decision Making: CDPs provide companies with a solid foundation for data-driven decision making. With accurate, up-to-date information, leaders can make strategic decisions that drive growth and operational efficiency. They also facilitate advanced RFM analytics and predictive marketing analytics.
In short, CDPs are much more than just data repositories. They are digital transformation catalysts that enable enterprises to navigate the complex customer data landscape through a unified, high granularity view, delivering competitive advantage by enabling deeper customer insights and enabling more meaningful and profitable interactions.
Fortunately, these aspects are measurable and their consequences are positive in terms of sales and profitability. It is true that many citations and studies are conducted by the software vendors themselves, and this means that the data should be taken with extreme caution. That a group of companies has been successful with a CDP, or that CDP as a technology has business impact methods does not guarantee its positive ROI on an organization.
An interesting case is Segment, who conducted a survey with Aberdeen, and in it found that the average impact of a CDP within business results included, “9.1 times higher growth in customer satisfaction, 2.9 times higher year-over-year revenue growth, 5.7 times higher revenue in average customer spend and 4.9 times higher cross-sell growth.”
(…) CDP users accomplish far greater year-over-year (YoY) growth across those KPIs. Specifically, they enjoy 9.1x greater annual growth in customer satisfaction rates (10.0% vs. 1.1%) and 5.7x greater annual increase in customer lifetime value (10.2% vs. 1.8%) — the latter defined as the change in overall client spend during the course of the relationship with that buyer
Aberdeen survey
There are many variables that can explain these results, and in fact the Aberdeen survey should not be taken as direct proof that CDPs will increase sales directly, but rather that a CDP directly influences the user experience, and it is the user experience itself that fosters increased sales.
Several articles mention this reality. Blake Morgan in Forbes mentions that due to the highly personalized environments we already experience (in Amazon or Netflix for example) leads us to expect that same level of personalization in other products and services. This article collects different interesting figures and studies in this regard. Accenture also points to the importance of personalization in its Personalization Pulse Check where they indicate that “91% of consumers are more likely to buy from brands that recognize them, remember them and provide relevant offers and recommendations“.
McKinsey endorses and finds more outstanding figures in more recent studies conducted in the aftermath of the pandemic. In their article “The value of getting personalization right -or wrong- is multiplying” they highlight that after the global COVID-19 crisis the need for personalization has only increased and increased its impact on sales: “71% of consumers expect personalization and 76% of them are frustrated when they don’t find it“.
Following this, there is a key idea to consider: only with the activation of high quality data can a level of personalization that has a positive impact on business be achieved. We cannot delude ourselves into the false premise that we can personalize “here and now” without having integrated, high quality data. This is probably where CDP has gained the most popularity, as it is a technology that reduces the technical gap in this particular aspect.
Only with high quality data activation can a level of personalization that has a positive impact on business be achieved
The above leads us to a scenario in which investing in MarTech and an adequate data infrastructure is necessary not only for a personalization process, but also for a CDP implementation process. Certainly a CDP is a tool that can help increase data quality and integrity (because of what was mentioned earlier in this section). But intrinsically a CDP can improve data to a limited extent, otherwise it would risk being just another data silo, rendering unlikely one of its main purposes: to break down silos.
Certainly there is a strong dependency between data quality and the actionability we get from a CDP. Without proper data quality the segmentations will not be of high quality, the single user view will have errors, privacy might not be respected and integrations might even be useless altogether.
1.2.1 Case studies
One of the best methods to understand the applicability and importance of CDP in business results is in case studies. There are multiple sources online where there are high quality case studies. The important thing about the use cases is to understand how to achieve a CDP implementation completely aligned with the business objectives, always having a careful view of the results, since being cases created by the software vendors and the same executives that hired them it is in their best interest to show positive results, that is, the creators of the use cases are in a clear conflict of interest.
This does not imply that reading use cases has no value, it just means that we should read them with a bit of skepticism. You can find detailed CDP case studies at:
- Tealium
- Treasure Data
- Customer Data Platform Institute
- Bloomreach
- Segment
- Adobe
- Hightouch
- Census
- Salesforce
A good example is the case of Albertsons, a major supermarket chain, in which they improved customer experience (CX) through ActionIQ (packaged CDP vendor). In this particular case ActionIQ was selected for its ability to collect, analyze and activate customer data on a large scale. The process had a strong customer data unification aspect along with effective orchestration of data flows to deliver personalized experiences and real-time communications.
Albertsons was able to develop unified customer profiles, combining transactional and behavioral data, resulting in a better understanding of customer intentions and preferences. This allowed them to activate more effective and faster marketing and loyalty campaigns, going from taking a month to implement one use case to being able to activate five in the same period.
The results achieved included a significant increase in conversion rates and average purchase value, with weekly click-through rates of approximately 30% and coupon redemption rates of 37%. This demonstrates the power of a well-implemented CDP to deliver omnichannel personalization and improve customer engagement maturity. Certainly personalization is the fundamental lever in the results of a CDP.
Another case study is that of Canva in which we are talking about a composable CDP implementation (Snowflake, Census, Fivetran and dbt). This case is striking because it includes from the outset the engineering team. An interesting challenge because with more than 125 million monthly active users, the technological solution to be applied was inevitably complex.
The results of this strategy pursued by Canva include greater personalization in messaging through more complex and faster segmentation by enabling data analysts to build more complex segments in minutes rather than weeks, using machine learning (ML) based segmentation models.
This case study highlights the benefits of the composable CDP, by managing to address specific challenges only existing in a service with so many active users and with a growing demand for personalization that had to be activated on platforms such as Facebook and Google Ads. This undoubtedly highlights the strengths of a composable CDP, as these same results would hardly be replicated by a packaged CDP.
In summary, Canva has achieved more effective message personalization, efficient segmentation and a more dynamic data feedback loop by implementing a CDP comprised of industry-leading tools.
Generally the case studies highlight business outcomes closely related to metrics specific to personalization and user experience as it is itself the main point of impact of a CDP.
1.2.2 Costs
CDP also impacts an organization at a cost level: it involves a considerable investment in technology, budget and time. If a CDP is not fully actionable without an optimal data infrastructure it is likely to bring a negative ROI in the organization. Therefore, having a CDP from which we can derive real benefits also involves a detailed roadmap backed by a business and data strategy.
Certainly the investment levels are not the same depending on the industry and the type of company, those that have massive data and also have not invested in a clean, scalable and high quality data structure are the ones that will have to invest a lot more.
The costs associated with implementing a Customer Data Platform (CDP) go beyond the direct investment in technology. To get the most out of a CDP, companies must consider several factors that involve both tangible and intangible costs.
- Data infrastructure: For a CDP to be effective, a solid and well-organized data infrastructure is required. This can involve significant investments, especially for those companies that do not yet have clean, scalable and high-quality data systems.
- Data-driven organizational culture: Developing a corporate culture that prioritizes and values data is crucial. This involves training employees in understanding and using data, which may require time and resources for trainings and changes in organizational mindset.
- Digital transformation: A CDP is often part of a broader digital transformation strategy. This means that the company may need to upgrade or change existing systems, processes and operations.
- Data management discipline: Establishing and maintaining rigorous data management discipline is critical. This includes implementing processes for data collection, cleansing, integration and analysis, which may require investments in specialized tools and personnel.
- Employee training and development: Expanding employees’ technical knowledge to work effectively with a CDP is another cost to consider. This can range from specific training in the use of the CDP to general education in data analytics and data-driven decision making.
These costs and efforts can be especially challenging for large organizations, where change and adaptation processes tend to be slower and more complex. In addition, these transformation and adaptation processes are not static, but require continuous engagement and adaptations over time to stay aligned with technology evolutions and business needs.
Therefore, it is crucial that companies not only consider the initial cost of implementing a CDP, but also the long-term costs and resources required to effectively integrate it into business strategy and day-to-day operations.
2. Background and Context
Customer Data Platforms are not a particularly old technology, in fact it is possible to argue that the CDP is nothing more than a stage of maturation of what has been a constant evolution of the technologies associated with customer information. Loyalty programs, rewards and customer databases could be considered as a primitive stage of CDP, not from a technological perspective (a points program has little or nothing to do with the technological infrastructure of a CDP) but from a conceptual perspective.
In any case, the truth is that the term CDP does not have an “exact” birth date. If we take Google Trends as a reference we could think that its “real” starting point is close to 2016. Same year in which the GDPR was announced.
Arguably the first to coin the terminology "Customer Data Platform" was David Raab (Founder of the Customer Data Platform Institute) in a blog entitled Customer Experience Matrix.
“Customer” shows the scope extends to all customer-related functions, not just marketing;
David Raab
“Data” shows the primary focus is on data, not execution; and
“Platform” shows it does more than data management while supporting other systems
To reach this term historically we have gone through different technologies that consequently have brought a constant need for processing and integration. The general idea that the history of the CDP is intrinsically tied to the evolution of other technologies is also exposed by The Customer Data Platform Resource who highlight an observable evolution in the last 20 years. And no wonder, in their article "The history of the CDP & CRM" they mention that the first CRM software (TeleMagic) in 1985. So we are talking about a technology whose foundations have decades of history.
Oracle offers a similar historical picture by mentioning again the existence of CRMs in the 1990s and the constant interaction between different tools that led to the emergence of CDPs such as: MDM (Master Data Management), DMP (Data Management Platforms), CIP (Customer Intelligence Platform), etc. However, Oracle's general idea is simple: "CDPs are born as a reaction to the need for an improved customer experience in an omnichannel environment".
In a simplified way we could understand everything as a relatively simple process: the CDP is an expected step at a historical level once organizations managed to mature the different IT systems in charge of storing and processing their data, the CDP is nothing more than the focal point where they converge, and therefore its history is tied to the evolution of the aforementioned technologies.
In the present year 2023 we see that the evolution of the CDP is being shaped mainly by privacy policies and the need to optimize marketing efforts, especially in an environment of financial stress and economic uncertainty. This implies that now CDPs as technologies still have a notorious level of travel, especially with the entry of generative Artificial Intelligences.
Now, the historical evolution of CDPs has another crucial factor and that is the increasing supply of MarTech technologies: since 2014 we have observed a notorious growth in the supply of all types of technologies. Certainly the birth of CDPs coincides in this commercial phenomenon that we are still experiencing to this day.
This leads us to think that CDPs are also associated with the growing market need to optimize processes through technology. This environment is undoubtedly one of the major catalysts for the existence of CDPs.
This puts CDP vendors under stress due to the highly competitive nature of the industry and may cause some of them to exaggerate the actual capabilities of their software. There is no way to state such a reality, but there is a record that a former Salesforce vice president reported that "Salesforce's claims about the real-time processing capabilities of its CDP were false". Certainly these unfortunate facts force us to take soberly the promises and results reported by any software vendor.
Privacy is the last piece in leveraging the current and historical development of CDPs by being precisely a technology that bases all data mining on customer information, which is directly connected to laws such as GDPR and the obsolescence of third-party cookies. As Didomi well shows in its privacy barometer, over time privacy laws and their enforcement has been increasingly forceful across the globe. Certainly these changes have had an impact on digital marketing, especially in data collection.
It should be clarified that all privacy policies and changes in the operation of browsers (such as the increasing privacy measures in Safari and Firefox or the upcoming obsolescence of third party cookies in Chrome) do not have a direct impact on the operation of CDPs, but rather strengthen the growing demand for their ability to activate First Party Data (which at the time of writing this guide, is positioned as one of the best methods to generate segmented audiences). What we can highlight is that data protection policies such as GDPR somehow shape the governance methods and techniques present in a CDP (and any other technology that treats personal data).
Finally, the evolution of CDPs could be influenced by the continued development of emerging technologies such as blockchain, which could offer new ways to manage and protect customer data. With these developments, CDPs will not only become essential tools for customer data management, but also key enablers for customer experience innovation and operational efficiency. Although this is somewhat speculative.
In conclusion, CDPs have come a long way since their inception, evolving from simple customer databases to sophisticated platforms that play a crucial role in marketing strategy and business decision making. As we move forward, continuous adaptation and innovation will be essential to stay ahead in the dynamic world of marketing and data management.
2.1 Evolution of the CDPs
The evolution of Customer Data Platforms (CDP) has been influenced by three key trends: the need for more efficient data integration and activation, increasing regulation around data privacy, and advances in technologies such as cloud computing, Machine Learning and Artificial Intelligence. These strands have shaped the development of CDPs towards more robust and sophisticated platforms.
Data integration and activation have become crucial aspects for companies seeking a deeper and more operational understanding of their customers as well as an optimization of their advertising investments through personalization and a better user experience. This is reflected in the way CDPs have evolved to offer data unification solutions, providing a holistic and accessible view of the customer.
In terms of privacy regulation, the evolution of CDPs has been heavily influenced by laws such as GDPR and CCPA. These regulations have driven the development of CDP features that ensure legal compliance while respecting user privacy and consent. This adaptation is essential to maintain customer trust and avoid legal penalties, which has led to CDPs incorporating more robust consent and privacy management functionalities.
Finally, advances in cloud computing, Machine Learning and Artificial Intelligence have opened up new possibilities for CDPs. These technologies enable platforms to analyze large volumes of data faster and more accurately, delivering deeper insights and more accurate predictions. Companies such as Adobe are investing in cloud marketing solutions that integrate generative AI to automate and enrich marketing campaigns and others such as Salesforce are already conceptually visualizing what this future will look like. Generative AI not only improves the personalization and effectiveness of campaigns, but can also predict consumer trends and behaviors, making CDPs even more powerful tools for marketers.
In itself we can summarize the advancement and evolution of CDPs in the following eras:
Pre-CDP
- 1990s - Launch of CRM: Development of Customer Relationship Management (CRM) systems, focused on interaction and information related to sales and services.
- Early 2000s - Emergence of DMPs: Emergence of Data Management Platforms (DMPs) for digital advertising, focused on online data.
Early CDP
- Mid-2000s - First Generation CDP: The first CDPs appear as solutions to unify customer data from multiple sources, both online and offline.
Modern CDP
- Late 2000s and Early 2010s - CDP Maturation: CDPs evolve with real-time data integration capabilities, advanced analytics and personalized marketing actions.
- Mid 2010s - CDP Expansion and Specialization: Expansion of CDP functionalities and specialization for different industries and needs.
Real-Time Marketing Centric CDP
- End of 2010 - Focus on Privacy and Compliance: Adaptation of CDP to regulations such as GDPR and CCPA.
- 2020 and Beyond - Era of Artificial Intelligence and Machine Learning: AI and ML integration for advanced segmentation, real-time personalization and behavior prediction.
- Today - CDP as the Central Axis of Digital Marketing and Customer Experience: Consolidation of CDP as key tools for digital marketing strategies and an integral vision of the customer.
2.1.1 The Rise of CRM and the Need for CDPs
The origins of CRM (Customer Relationship Management) go back to the 1980s, with figures such as Robert and Kate Kestnbaum, pioneers in database marketing. This approach statistically analyzed customer databases to identify those most likely to respond to marketing campaigns. In 1986, ACT! software marked a milestone in contact management, being a precursor to modern CRM, by enabling efficient organization and storage of customer contact information.
During the 1990s, the CRM industry experienced significant growth. Sales force automation (SFA) integrated features of database marketing with contact management, providing companies with more detailed customer information. In this period, Tom Siebel, with Siebel Systems, became a leader in the SFA market, evolving into what we now know as CRM software.
In the late 1990s and early 2000s, the CRM industry witnessed significant changes, with the entry of major players such as Oracle and the emergence of Salesforce, which introduced the Software as a Service (SaaS) model and marked the beginning of cloud-based CRM solutions. However, in 2006, more than 50% of CRM installations were considered failures, according to Gartner, reflecting significant challenges in implementation and effectiveness.
Faced with the limitations of CRM, especially in managing and analyzing large volumes of data from multiple sources, the need for more advanced and flexible platforms began to emerge. This is where Customer Data Platforms (CDPs) emerge, designed to connect and organize customer data from diverse sources, including CRMs, content management systems (CMSs) and digital asset management solutions (DAMs).
It is common to think that CDPs are nothing more than a "modified CRM" or it is complex in some cases to differentiate their role and inherent technical differences. The truth is that CDPs exist to overcome challenges where CRMs have struggled such as synchronization, mapping and data transfer. That is, it is not as if it could not be possible to integrate all customer information into a CRM in the same way as it is done with a CDP, the trade-off will almost inevitably be that due to the technical design of the CRM we would have much higher data latency, inconsistencies between databases and very high computational cost.
Given these limitations of CRMs, the tools we know today as CDP were born, which as explained at the beginning of this guide, their purpose is based on the activation and integration of data, something that is far from the purpose of CRMs: to manage customer relationships.
2.1.2 The Transition to a Cookieless Future.
We have experienced in recent years a growing increase in public interest in online privacy, not only because of the emergence of laws such as the GDPR, but also because of initiatives that organizations such as Mozilla through its Firefox browser, in which initiated the blocking of third-party cookies from 2019, leading Google to confirm the same with its Chrome browser in 2020.
Certainly cookies have played a fundamental role in the development of the internet, and in particular in the way advertising services operate, so much so that the announcement of the end of third-party cookies supposed an existential threat to data providers.
The operation of cookies, from a very reduced and simplified explanation, can be understood as an information exchange mechanism where an external website that a user visits can measure and personalize the user's interaction. For example, if a user visits a website with puppy videos and this website has Google scripts, it would have the opportunity to know which user is and assign to his profile "the taste for puppy videos". Likewise it could read other attributes of the user to serve him advertising, for example, "see that said user has searched for washing machines online recently" and therefore serve him personalized ads. This behavior can be visually summarized in the following diagram:
Of course, with the inevitable end of third-party cookies these personalization and measurement capabilities will disappear and will have to be replaced by other technologies. Google has its own initiatives in The Privacy Sandbox, as well as Xandr (Microsoft) and Meta.
Beyond the technical details, what is certain is that different expert voices point out that the exploitation of First Party Data is going to be key to finding success in this cookieless future. It is precisely here where CDPs show their advantage since being tools with a clear 360 view of an organization's customers they are positioned as the fundamental tool as a replacement to cookies, at least from the perspective of digital advertising.
The big difference is quite simple, instead of relying on a cookie and the browsing history of a user, through a CDP there is an export of personal data (email, name, address, phone number, etc.) to advertising platforms (Meta, Google, TikTok, Microsoft, etc.) and these will be responsible for determining which users of its platform are part of that audience.
Segment Creation
'Customers with Birthdays This Month'] --> export[Export of Personal Information] export --> GoogleAds[Google] export --> Meta[Meta] export --> TikTok[ByteDance] export --> Microsoft[Microsoft] Meta -->|Personal Data Matching| Newadsaudience1[New Audience on Meta] Microsoft -->|Personal Data Matching| Newadsaudience2[New Audience on Microsoft] GoogleAds -->|Personal Data Matching| Newadsaudience3[New Audience on Google Ads] TikTok -->|Personal Data Matching| Newadsaudience4[New Audience on ByteDance] Newadsaudience1 -->|Share| Audience[Audience Available for Advertiser] Newadsaudience2 -->|Share| Audience[Audience Available for Advertiser] Newadsaudience3 -->|Share| Audience[Audience Available for Advertiser] Newadsaudience4 -->|Share| Audience[Audience Available for Advertiser]
A CDP is certainly not the only solution in a cookieless future, but it does play a key role in segmenting and personalizing the user experience.
2.1.3
User Privacy and Data Consent
User privacy and data consent are critical aspects in the management of Customer Data Platforms (CDP) being an element of legal compliance required to process and activate personal data. Without active consent a CDP loses its purpose. Specifically in digital marketing, increasing regulatory pressure has been experienced that makes it more difficult to obtain consent.
- Evolution of Regulations: Rules and regulations on data privacy and its use are constantly evolving and tend to become more stringent. International privacy regulations have established severe penalties for companies that fail to properly collect and manage consumer data. NOYB is one of the most prominent organizations in the fight for user privacy and its website perfectly captures a history of penalties and legal changes.
- Informed Consent: Managing consent means giving users greater control over what data is collected and how it will be used. This goes beyond a simple opt-in or opt-out; specific consent questions should be asked for each function, such as marketing or customer service, and tailor data collection to user preferences.
- Regulatory Compliance: Consent management is crucial today because it records and tracks consent collection, helping organizations comply with global privacy regulations. While customer data collection and management are necessary for marketing and advertising campaigns, organizations must do so in compliance with local and international data privacy laws.
Considering the above, multiple consent management technologies have emerged, or mechanisms to integrate consent into a CDP. A good example is the case of Tealium, who has decided to integrate it natively into its CDP solution.
To manage customer data while respecting privacy laws and collecting user consent, it is advisable to combine CDP with a consent management platform to unify consent data with customer data collected on different platforms. Alternatively, opt for a CDP solution that solves this specific casuistry.
CDPs have an advantage in this regard: their ability to create a unified customer profile across channels and platforms facilitates the fulfillment of data requests and preferences. Some CDPs are able to integrate with consent management platforms, becoming the trusted repository of user data and ensuring compliance across all connected systems.
On the other hand to implement an effective personalization strategy using CDP technologies, it is critical to have a high consent rate and robust reconsent strategies. The nature of user consent in the context of CDPs is dynamic and requires a continuous approach to keep it relevant and valid by following these principles:
- Consent as the Basis for Personalization: User consent is a key issue, as traditional personalization methods based on third-party cookies and browser tracking are no longer compatible with regulations and consumer demands. Respecting consent is crucial to maintaining brand integrity and revenue growth. Future personalization requires organizations to be flexible enough to adapt to consumer demands through varied approaches that are tailored to each individual.
- Encourage Zero-Party Data Capture: An effective strategy for obtaining active consent is to focus on zero-party data capture as much as possible. Brands that provide value to their customers in exchange for their data can actively obtain consent along with valuable information about their target market. This can be achieved through customer satisfaction surveys, questionnaires, personalized profiles and loyalty programs.
- Customer Engagement and Brand Loyalty: In addition to capturing consent and obtaining direct consumer feedback, this approach focuses on customer engagement, which simultaneously increases brand awareness and loyalty. "No one is going to give up their personal data for nothing in return."
- Obtain Consent on a Continuous Basis: The second strategy is to obtain consent as frequently as possible. This is only possible through continuous interaction with each customer, a high quality of customer service and always providing something of value that justifies precisely that transfer of personal data. For example: "I feel comfortable giving my data to this company because with it I get an additional 5% discount."
2.2 Current Market Scenario
The CDP market size is projected to grow significantly from $2.31 B in 2023 to $7.91 B in 2028 according to a report by Mordor Intelligence. The growing need for omnichannel experiences, which adopt a customer-centric strategy to enhance the user experience, is driving the market expansion. In addition, the real-time and personalized data analytics offered by CDPs is fueling this growth, providing organizations with a more meaningful understanding of consumer behavior to implement their marketing plans more efficiently.
Also, according to this report, the CDP market is fragmented by a multitude of competitors, which drives constant innovation, product improvement and the implementation of customer acquisition and retention strategies. In an ever-growing market, it is crucial for marketers to have a broad customer acquisition capability at a time like the present.
This is combined with an inflationary environment in 2023 that puts pressure on maintenance and development costs.
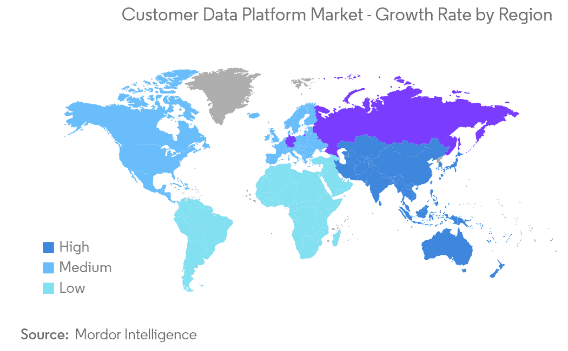
2.2.1 Market Figures
- CDPs will reach a market size of $7.91 billion with a CAGR of 27.91%. Asia Pacific being the fastest growing region and North America the largest market. Other research even estimates that its market will be $19.7 billion in 2027.
- The main players in the market are SAP, Oracle, Segment, Adobe and Salesforce.
- According to Mordor Intelligence, the healthcare sector will be primarily responsible for the growth of the CDP market.
- The CDP market is a market that tends to be fragmented, so there are many alternatives, which means that no one company dominates the market.
- The popularization of CDPs will occur in part thanks to the availability of Artificial Intelligence and Machine Learning according to Fortune Business Insights.
In addition to this, the market report presented by the CDP Institute brings us a number of important insights:
- The industry has grown from 1,264 employees in 2016 to 15,132 in 2022 according to . A growth of more than 10 times in less than 10 years.
- Continued Expansion: Industry revenues for 2022 are expected to reach $2 billion, representing a 25% increase over 2021.
- Strong Growth in Large Companies: The top 25% of suppliers experienced an average growth of 13%, compared to 4% for all others. This group also increased its share of financing and the average number of employees per company.
- Challenges in Europe and Stability in APAC: While U.S.-based suppliers expanded their share of business, employment and financing, European suppliers saw their share decline to the lowest levels ever recorded, reflecting slow adoption in EMEA markets and the strong presence of U.S.-based suppliers in some of those markets. The industry share of APAC suppliers remained roughly stable.
Finally, the last questionnaire to CDP Institute members tells us about several aspects to consider:
- Reduction in Data Unification: There was a decrease in the number of respondents reporting having a unified customer database or CDP in 2023 compared to 2022. This is the first time the numbers have decreased since the survey began in 2017.
- Cost Pressures: Budget pressures are cited as a barrier to better utilization of client data, with a significant increase in martech selection based on initial and operational cost.
- Focus on Costs and Satisfaction: Companies that focus on costs are generally less satisfied with their martech investments compared to those that select based on features.
- Improvement in CDP Implementation Success Rates: The proportion of CDP implementations that are generating significant value increased from 61% to 80%, the highest level on record.
- Increased Investment in Privacy Compliance: A record 27% of companies actively promote their privacy policies as a benefit to consumers, up from 21% in the previous report.
In other words, CDPs are growing, with ample budgetary pressure, which in turn is accompanied by more and better results. A higher level of innovation and investment is expected from the various players in the market.
2.3 Breaking Data Silos
In the context of Customer Data Platforms (CDP), breaking down data silos is crucial to achieve a holistic view of the customer and maximize the effectiveness of marketing strategies and customer experience. Customer data, which includes personal, behavioral, psychological or demographic information collected during their experience with the organization, is often stored in silos within data (e.g., their purchases are in the ERP and their contact details are in the CRM), leading to problems such as duplicate entries, outdated or incorrect information, and lack of collaboration and transparency.
In the context of a CDP when we talk about "breaking down silos" we are referring specifically in the unification of data where the linking key is customer identifiers. After all the role of a CDP is precisely in unifying all available information on each customer.
Breaking down data silos is a process in itself. As Deloitte points out in its article Breaking Down Data Silos: detecting where data is stored, how it is being collected, how relevant it is and how up-to-date it is.
We certainly need to add one more aspect to this idea, and that is recognizing data integration barriers, be they technical or legal. This particular issue can be a fundamental challenge in data integration within a CDP.
Certainly CDPs (particularly packaged ones) facilitate the unification of information by having specific technologies that unify the view of customers through an identity resolution service, which effectively eliminates any silos. Although this implies that the data and identifiers are of high quality and trust.
Benefits of Eliminating Data Silos:
- Holistic Customer View: By breaking down silos, companies can gain a complete and unified view of their customers, which improves decision making and personalization of interactions.
- Improved Operational Efficiency: Elimination of duplication and improved information flow between departments leads to greater operational efficiency.
- Innovation and Business Agility: Data integration fosters innovation and agility by enabling organizations to respond quickly to changing market demands and customer preferences.
Challenges and Considerations:.
- Change Management: Breaking data silos often requires a change in organizational culture and business processes.
- Privacy and Security Compliance: It is important to ensure that data integration is performed in compliance with privacy laws and information security practices.
- Normalize information: Data is not always accessible or formats are completely unified, which may involve additional data engineering work.
- Latency: On many occasions batch updates can generate unexpected latencies that can affect the correct integration of data.
2.3.1 Importance of a 360° Customer View
The 360 customer view is a holistic perspective that amalgamates all relevant customer data to provide a deep, multidimensional understanding. This view is vital in today's era, where personalization and precision in marketing are key to business success.
A 360 view of the customeris fundamental to modern marketing, as it transforms the customer relationship from transactional to experiential, laying the foundation for long-lasting relationships and positive endorsements. By analyzing and understanding the various aspects of customers, companies can deliver more personalized experiences, aligning the efforts of different departments and ensuring consistency across all interactions.
This holistic view captures each customer's interaction history, mapping results for each event, allowing you to predict behaviors and trends, and understand the reasons behind key actions such as shopping cart abandonment or attrition. Comprehensive data collection leads to more accurate persona planning and uncovering broad trends and user responses.
In addition to being a mechanism for capturing and unifying data, it also facilitates its activation, transforming it into valuable and actionable insights. By unifying more data from each customer, more detailed and accurate information can be inferred, allowing the creation of more specific audience segmentations and personalized experiences. This wealth of data is invaluable to marketing teams, providing a solid foundation for strategic decision making, from product development to targeted marketing campaigns.
The 360 view is what enables sophisticated activation across journeys, audiences and segments. It is the way to cultivate long-lasting, high-value relationships with each and every customer.
Another fundamental aspect lies in the importance of ensuring that the data is actionable and useful. This involves ensuring that data is in consistent formats, up-to-date and easily accessible to the relevant teams. This accessibility and usability of data enhances a company's ability to respond nimbly to changing customer needs and behaviors, thereby improving customer satisfaction, loyalty and, ultimately, marketing and sales ROI.
3. Technical Basics of CDPs
CDPs have a fundamental technical structure that allows them to fulfill 6 functions: data integration, unification of customer profiles, segmentation and analysis, data distribution to other platforms, compliance with legal regulations and finally interface and usability. In order for these functions to become a reality, they must comply with a series of technical fundamentals.
The first foundation is data flow and integration, every CDP must have the ability to integrate data from various sources whether they are customer relationship systems such as CRM or real-time interaction information (website interactions). Generally every CDP has two methods under which they achieve this:
- APIs and Webhooks: CDPs often integrate with other platforms and systems via APIs and webhooks, enabling dynamic, real-time data transfer.
- ETL (Extract, Transform, Load): CDPs use ETL processes to extract data from various sources, transform it into a consistent format and load it into the CDP database.
Under these methods CDPs get access to data from any system and will therefore be able to integrate the necessary data for processing. For example, a CDP could apply an ETL to extract sales from the ERP every day at midnight or implement a webhook that allows the web server to send the interactions that each customer makes in e-commerce.
Technicalities aside, it is important to emphasize that the above examples open the way to another technical rationale, which is that processing sales made every 24 hours is not the same as receiving real-time user interactions, so CDPs also take into consideration two types of methods under which they process data:
- Batch Processing: CDP uses batch processing to import and process large volumes of data accumulated over a period of time. This method is efficient for historical data and does not require real-time processing.
- Stream Processing: For real-time data, CDPs implement stream processing. This is crucial for capturing and responding to real-time customer interactions, such as behavior on the website or in mobile apps.
These methods are crucial for combining and organizing data from various sources, creating complete and detailed profiles of each customer. These unified profiles are formed using advanced techniques that identify and relate different pieces of information. However, it is important to mention that not all data is processed instantaneously. Some audiences are updated according to the batch processing cycle, which means that certain CDP functions do not operate in real time and may present certain delays in the availability of information.
The second foundation is data storage. Data warehousing in a CDP is a crucial aspect, as it acts as the central core where all collected and processed data are congregated. In the case of a composable CDP the choice of a suitable data warehouse is fundamental, as it must be able to handle not only a large amount of data, but also various types of data, from structured to semi-structured and unstructured. For the case of packaged CDPs this problem is usually solved in itself and is a technical challenge overcome.
Some CDPs use data lakes in combination with data warehouses. While data warehouses store already processed and structured data, data lakes can contain data in its raw form, allowing for deeper analysis and the possibility of discovering insights that would otherwise not be evident. This combination allows CDPs to be more flexible and powerful in terms of data analysis.
In addition, data storage in a CDP must be secure and comply with all relevant regulations, such as GDPR in Europe or CCPA in California. This implies having robust access controls, data encryption and mechanisms to ensure the privacy and security of customer information. This implies additional developments and technologies in the technological structure of the CDP since data must comply with levels of restriction and encryption specific to each region or regulation. This implies that in fundamental terms a CDP must be secure, private and with strong data governance tools.
After data collection and storage, the next technical foundation is the unification of customer profiles. CDPs must have advanced algorithms to identify and unify a customer's data across different touchpoints and platforms. This involves resolving identities, where disparate data is linked to a single customer profile, providing a 360-degree view of the customer. Proprietary or open source solutions are often available here. It is possible to find different solutions on the market, although again, this tends to be a problem that packaged CDPs already have solved, it will be in the case of composable CDPs where libraries, services or even proprietary developments must be sought in order to implement this technical foundation.
With unified profiles, CDPs can perform advanced segmentation and detailed analysis. This includes the ability to segment audiences based on a variety of criteria, such as behavior, demographics, transactions, and more. Analytics can range from simple reporting to predictive modeling and machine learning to anticipate future customer behaviors or identify high-value segments.
Finally, the interface and usability of a CDP are critical to its adoption and effectiveness. The platform must be intuitive and easy to use, allowing non-technical users to access and operate the tool efficiently. This includes customizable dashboards, easy-to-interpret reports and data visualization tools that facilitate data-driven decision making.
The technical underpinnings of a CDP range from data integration and processing to data storage, security, customer profile unification, segmentation, analytics and the user interface. Each of these elements plays a crucial role in the effective operation of a CDP, enabling companies to gain a deeper understanding of their customers and significantly improve their marketing and customer experience strategies.
3.1 Technological Architecture
The technology architecture of any customer data platform (CDP) is comprised of several key components that work together to collect, process, store and analyze customer data. At the core of a CDP is its ability to integrate data from multiple sources. These sources include first-party data such as website interactions, CRM and ERP data, as well as data from marketing tracking and point-of-sale systems. In addition, CDPs can incorporate second- and third-party data, which comes from external data brokers, social media platforms such as Meta and Google, and ad servers. This data integration is crucial to providing a complete and unified view of the customer, enabling companies to better understand their behaviors and needs.
Once collected, this data is processed and stored in a centralized data warehousing system within the CDP. This process involves identity resolution, where data from different sources is brought together to create unified customer profiles. Data processing can include data modeling, where models are created for predictive analytics and personalization. The ability to process and model data enables CDPs to perform advanced segmentation, cohort analysis and calculate customer lifetime value (CLTV). In addition, the CDP architecture must ensure security and regulatory compliance, especially with regard to customer data privacy. Finally, CDPs distribute this processed data to various destinations, which may include marketing platforms for personalization and audience segmentation, as well as business intelligence and data science tools for more detailed analytics and reporting. This end-to-end architecture enables companies to maximize the value of their customer data, improving decision making and optimizing marketing and customer service strategies.
The architecture itself is different depending on the CDP typology.
3.1.1 Composable CDP
Composable CDPs are characterized by their layered structure of services that operate directly on top of an organization's data warehouse, offering significant flexibility and customization compared to packaged CDPs. These service layers can include data ingestion and processing, identity resolution, data modeling, and advanced segmentation, among others. Each of these layers is designed to work with the data stored in the data warehouse, allowing enterprises to maximize their existing data assets. This configuration offers a great advantage in terms of adaptability, allowing organizations to customize their CDP to meet specific needs, such as meeting particular data compliance requirements, integrating unique data sources, or developing specific analytics capabilities.
However, composable CDPs also present unique challenges, especially in terms of infrastructure complexity and management. They require considerable investment in terms of configuration and maintenance, as service layers must be configured and managed to work harmoniously with the data warehouse. In addition, integrating various data sources into a composable CDP can be a more complex technical process, requiring specialized expertise in data architecture and integration systems. The flexibility of these systems also means that the responsibility for ensuring security, performance and scalability rests largely with the organization. Therefore, while composable CDPs offer significant customization and control, they also require a greater investment in technical and human resources to ensure their effectiveness and efficiency.
3.1.2
Packaged CDP
Packaged CDPs, being more closed and preconfigured solutions, present unique technical challenges compared to composable CDPs. Although they minimize the need for complex infrastructure work, the main challenge lies in effectively integrating and managing the duplication of data from various sources. In these structures, the ability to integrate seamlessly with existing systems, such as CRMs, ERPs and digital marketing platforms, is crucial. The frequency and efficiency of these integrations play a vital role in maintaining data consistency and real-time or near real-time updating, which is essential to ensure that insights and actions derived from the CDP are relevant and timely.
In addition, managing data quality in a packaged CDP is another significant technical challenge. With data coming from multiple sources, it is essential to have robust mechanisms for data cleansing, normalization and deduplication, thus ensuring the accuracy and usefulness of the stored information. This process is critical to ensure that the insights generated are reliable and can be used effectively for decision making and personalized marketing strategies. Therefore, while packaged CDPs offer the advantage of easier implementation and maintenance, they require meticulous attention to data integration and data quality management to maximize their effectiveness.
3.2 Subtypes of CDPs according to Functions
According to the CDP Institute, there are several types of CDPs according to the different functionalities in which they specialize. In this sense, the trend seems to be that all these functions are present in almost any CDP and therefore it seems that this definition will be less and less fuzzy in the future. However at present it is an effective method to understand which CDP technology to hire based on the needs of the organization.
3.2.1 Data CDPs: Approach and Applications .
Data CDPs are primarily focused on the collection and unification of customer data from multiple sources. This type of CDP specializes in the effective integration of first-, second- and third-party data, ensuring that information is accessible and usable in a standardized format. They are ideal for organizations seeking to consolidate dispersed data into a single centralized repository, facilitating a holistic view of the customer. Data CDPs are critical for companies starting their data journey, providing the necessary infrastructure for data cleansing, normalization and enrichment, preparing them for more complex analysis and segmentation.
3.2.2 Analytics CDPs: Data Analysis and Utilization .
Analytics CDPs take the functionality of CDPs a step further, providing advanced data analysis and visualization tools. These CDPs not only collect and aggregate data, but also offer capabilities for deep dive analysis of customer behaviors, predictive segmentation and propensity modeling. They are particularly useful for companies looking to gain actionable insights from their customer data, such as identifying buying trends, forecasting future customer needs or better understanding interaction patterns. Analytics CDPs are valuable for marketing and sales teams that need advanced analytics to inform and optimize strategies and campaigns.
3.2.3 Campaign CDPs: Campaign Automation and Personalization .
Campaign CDPs focus on the automation and personalization of marketing campaigns. Integrating advanced segmentation and marketing automation capabilities, these CDPs enable companies to design and execute highly personalized campaigns based on unified customer data. These systems are ideal for omnichannel campaign execution, enabling marketers to create consistent, personalized customer experiences across multiple touch points. In addition, Campaign CDPs often include functionality for real-time campaign management and optimization, improving the effectiveness of marketing communications and increasing ROI.
3.2.4 Delivery CDPs: Delivery of Customer Experiences.
Finally, Delivery CDPs focus on the delivery and management of customer experiences across multiple channels. These CDPs integrate customer data with experience management systems, such as e-commerce platforms, mobile applications and content management systems. The primary goal is to use unified data to inform and enhance direct interaction with customers, ensuring that every touchpoint is relevant and engaging. Delivery CDPs are crucial for companies looking to improve the customer experience in real time, tailoring and personalizing interactions based on behavioral data, preferences and interaction history.
4. Implementation of a CDP
A CDP implementation project is an extensive and complex process that tends to take between 4 to 12 months depending on the technical complexity and the type of CDP to be implemented, with composable CDPs tending to take considerably longer. In general, this implementation process consists of the following phases:
- Martech Ecosystem Analysis.
- Evaluation of the organization's current marketing technology environment.
- Identification of existing tools and how they are integrated.
- Gap analysis and opportunities for CDP integration.
- Strategy and Definition of Use Cases.
- Development of a clear strategy for CDP implementation.
- Definition of specific use cases to guide CDP configuration and customization.
- Alignment of CDP strategy with overall business objectives.
- Benchmark and Technology and Supplier Selection.
- Comparison of different CDP solutions available on the market.
- Vendor evaluation based on criteria such as features, scalability, support and cost.
- Selection of the technology and suppliers that best fit the needs and objectives of the company.
- Technical Architecture Design.
- Creation of a detailed technical design for the implementation of the CDP.
- Planning the integration of the CDP with other systems and data sources.
- Ensuring that the architecture complies with data security and privacy standards.
- Work Plan.
- Preparation of a detailed implementation plan, including timeline and resource allocation.
- Establishment of key milestones and assignment of responsibilities.
- Preparedness for risk and contingency management.
- Kick-off and Start-up.
- Official start of the project with all interested parties.
- Final review of the work plan and confirmation of roles and responsibilities.
- Establishment of communication channels and follow-up processes.
- Implementation.
- Execution of CDP installation, configuration and integration activities.
- Continuous testing to ensure system functionality and performance.
- Adjustments and optimization based on test results and feedback.
- Application of First Use Cases.
- Practical implementation of the use cases defined during the strategy phase.
- Monitoring and evaluation of CDP performance in real scenarios.
- Compilation of lessons learned and adjustments needed to optimize the use of the CDP.
- Change Management and Digital Transformation.
- Active management of organizational change to facilitate the adoption of the CDP.
- Training and empowerment of users to maximize the use of the CDP.
- Fostering a data-driven culture to support digital transformation.
- Knowledge Transfer.
- Ensure that knowledge and competencies on the use of the CDP take root in the organization.
- Development of training material and documentation.
- Planning for ongoing support and evolution of the CDP system.
This could be a generic reference for a CDP implementation work plan of approximately 6 months duration:
4.1 Strategic planning and use cases
Successful implementation of a Customer Data Platform (CDP) starts with careful planning and strategy as this is what enables the use cases, fundamental to any CDP implementation. This involves identifying key business objectives, understanding the organization's specific data needs, and determining how CDP can help achieve these objectives. Planning should include defining the scope of the project, identifying key stakeholders, and allocating resources. It is essential to establish clear and realistic KPIs to measure the success of CDP implementation and ensure that all stakeholders have a clear understanding of the expected benefits and potential challenges.
It is clear use cases and a strategy that justifies the activation of a CDP that enables a successful implementation process. It is common for organizations that omit this part of the process to find CDP implementations that lack purpose, and therefore represent a negative ROI for the organization. Therefore, at a strategic level, these aspects are fundamental:
- Alignment with Business Objectives:
- Strategic planning ensures that CDP implementation is aligned with the organization's overall goals and objectives.
- It allows you to identify how CDP can support business initiatives, such as improving customer experience, increasing marketing efficiency or driving sales.
- Clear Requirements Definition:
- The use cases detail the specific needs that the CDP must satisfy, which helps to define the technical and functional requirements of the system.
- They provide a framework for prioritizing CDP features and functionality according to their relevance to business objectives.
- Customization and Configuration Guide:
- The definition of use cases guides the customization and configuration of the CDP, ensuring that it is tailored to the unique needs of the organization.
- It facilitates the integration of the CDP with other systems and data sources, ensuring that data flows are efficient and effective.
- Establishment of Expectations and Success Metrics:
- Strategic planning helps set clear expectations among all stakeholders about what the CDP will achieve.
- It allows defining specific metrics and KPIs to measure the success of the CDP implementation and the impact on business objectives.
- Basis for Change Management and Adoption:
- Well-defined planning facilitates organizational change management by providing a clear roadmap for CDP implementation and adoption.
- Helps communicate the value of the CDP to end users and gain their commitment and participation.
4.2 Selection of Technology and Suppliers
Selecting the right technology and vendors is a crucial step in implementing a CDP. Organizations should evaluate different CDP solutions based on their technical capabilities, ease of integration with existing systems, compliance with data privacy regulations, and support and services offered. The choice should align with the specific needs of the business and its long-term data strategy. It is advisable to conduct a thorough evaluation of vendors, including requests for proposals, product demonstrations and customer references.
It is important to consider that not all CDP software will suit all organizations. The needs and capabilities of each can vary considerably and therefore it is essential to take an agnostic view. To do this a good starting point is to evaluate the following aspects:
- Cost-Benefit Analysis:
- Perform a detailed cost-benefit analysis for each CDP solution considered. This includes not only the initial cost, but also the long-term costs related to operation, maintenance and upgrades.
- Evaluate the expected return on investment (ROI) based on the tangible and intangible benefits that each solution can bring.
- Evaluation of Scalability and Flexibility:
- Consider the scalability of CDP solutions to accommodate growth and changes in organizational needs.
- Evaluate the flexibility of the platform to adapt to new data sources, changes in data privacy regulations and evolving marketing strategies.
- Compatibility with Existing Infrastructure:
- Examine how the CDP solution integrates with existing technology infrastructure, including other Martech systems, CRM, ERP and data analytics platforms.
- Consider the need for possible upgrades or modifications to existing systems to achieve efficient integration.
- Support and Training:
- Evaluate the quality of support and training services offered by CDP providers.
- Consider the availability of learning resources, technical assistance and post-implementation support.
- Experience and Reputation of the Supplier:
- Investigate the supplier's track record and reputation in the market.
- Consult references and case studies from existing customers to better understand the effectiveness and reliability of your solutions.
- Focus on Data Security:
- Ensure that the CDP solution meets the highest data security standards.
- Evaluate the vendor's policies and practices regarding data security, including encryption, access control and compliance with regulations such as GDPR or CCPA.
- Testing and Evaluation Process:
- Consider conducting a pilot test or in-depth evaluation of the solution prior to full implementation.
- Use the testing phase to gather feedback from key users and validate the functionality and performance of the CDP.
4.3 Phases and Best Practices for Implementation
The implementation of a CDP can be divided into several phases: data preparation, integration, configuration and deployment, and finally, adoption and optimization. During the preparation phase, it is critical to ensure that the data is clean, complete and compliant. In the integration phase, connections to internal and external data sources must be established. Configuration and deployment involves customizing the CDP to meet specific business needs and ensuring that it works properly. Adoption and optimization are ongoing, requiring user training, performance monitoring and adjustments as needed. Best practices include involving end users from the beginning, ensuring data quality, and fostering a data-driven culture.
Perhaps one of the critical aspects is the selection of team leaders responsible for the correct execution of the project since a CDP being a cross-cutting technology in an organization has the risk of falling back on too many interlocutors, which brings the risk of preventing a correct development of the project either by faulty implementations due to an absence of strategic north or by a constant delay due to a lack of coordination between teams.
In addition, the implementation of a CDP is a process that may reveal the need for organizational and business process changes. Therefore, effective change management is crucial to ensure the adoption and long-term success of the CDP. This involves not only training users on new technology, but also helping them adapt to new processes and ways of working with data. Ongoing collaboration and engagement with all stakeholders, from senior management to end users, is essential to overcome resistance and foster a data-centric culture. In summary, an effective implementation of a CDP requires a balanced combination of technical skills, clear leadership, effective change management and cross-departmental collaboration.
5. Impact and Return on Investment (ROI) of CDPs
Measuring the impact of a Customer Data Platform (CDP) can be done through a variety of metrics and KPIs (Key Performance Indicators). These indicators should be aligned with the organization's strategic objectives and may vary depending on the specific use of the CDP. Some of the most relevant metrics and KPIs include:
- Improved Data Quality:
- Level of completeness and accuracy of customer data.
- Reduction of duplicate or inconsistent data.
- Operational Efficiency:
- Reduction in time and resources required for data management.
- Increased processing speed and access to data.
- Customer Engagement:
- Increased customer interaction, measured through metrics such as clicks, email opens, and time spent on the website.
- Improved conversion rates in marketing campaigns.
- Content Customization and Relevance:
- Increased effectiveness of personalized campaigns.
- Improved response and engagement rates in targeted campaigns.
- Customer Retention and Loyalty:
- Decrease in the churn rate (customers who stop using the services).
- Increase in purchase frequency or participation in loyalty programs.
- ROI of Marketing Campaigns:
- Increased return on investment in marketing campaigns.
- Improved ad spend efficiency and cost per acquisition (CPA).
- Segmentation and Targeting:
- Effective customer segmentation for marketing campaigns.
- Accuracy in targeting based on unified data.
- Fulfillment of Strategic Objectives:
- Contribution of the CDP to the achievement of long-term strategic objectives.
- Alignment with key initiatives such as digital transformation or customer experience.
- Internal User Satisfaction and Experience:
- Ease of use and employee satisfaction with the CDP.
- Improved data-driven decision making.
These metrics and KPIs should be monitored regularly to assess the impact of the CDP and adjust strategies as needed. It is critical that the KPIs selected reflect the specific business objectives and the role of the CDP within the organization's overall data strategy.
6. Glossary of Terms
Cookieless: This is a reference to the absence of measurement through the cookie system. For example: A server-to-server measurement system is a cookieless measurement mechanism.
First Party Data: First Party Data refers to information collected directly by an organization from its customers or users, including behaviors, interactions and transactions, without intermediaries involved. For example: The purchase history of each of the customers of an e-commerce.
Second Party Data: Data that is collected directly by one entity but shared or purchased by another entity. It is first party data in a different context and is often used to supplement a company's own data.
Third Party Data: Data collected by entities that do not have a direct relationship with the user from whom the data is collected. This data is purchased from data aggregators or other organizations and used to extend the scope or improve customer understanding.
ETL (Extract, Transform, Load): Process where data is extracted from various sources, transformed to fit requirements and loaded into a destination such as a data warehouse or CDP.
Reverse ETL: Reverse ETL process where data is extracted from a centralized system such as a data warehouse and sent back to operational or customer contact systems for marketing or personalization actions.
API (Application Programming Interface): Programming interface that enables interaction between different software systems, facilitating integration and data exchange.
Machine Learning: Branch of artificial intelligence that focuses on the development of systems capable of learning and improving from experience, without being explicitly programmed.
GDPR (General Data Protection Regulation): European Union regulation that regulates the processing and free movement of personal data, establishing guidelines for the collection and processing of personal data.